Decrypting Neurodegeneration
- Jeanette Luna
- Jan 30
- 11 min read
by Janet Yue Liu
art by Noelle Biehle
It all started during my time as a volunteer at a nursing home for residents afflicted with dementia. As soon as I walked in, I was struck by the silence that enveloped the facility. The residents seemed to exist in a limbo between contemplation and stupor, their eyes half-closed.
Among the patients, an elderly woman in the courtyard particularly moved me. When I approached her, her daughter shared their frustrating journey through multiple failed medications, each losing effectiveness as the disease progressed. This encounter crystallized a devastating truth: despite Alzheimer's being a leading cause of death, we still lack treatments that can halt its progression. Their struggle ignited my determination to explore truly effective therapies, not just temporary solutions.
Current Alzheimer's treatments have significant limitations, and diagnostic methods often detect the disease only after brain damage has occurred [1]. Innovative approaches are very much needed - and artificial intelligence (AI) may hold the key [2]. AI's ability to analyze complex datasets and identify patterns too laborious to the human eye could transform Alzheimer's research, from early detection to drug discovery.
Understanding Alzheimer's Disease
Alzheimer’s disease (AD) is a neurodegenerative disease that currently has no cure. In people over 65 years old [3, 4], AD is the most common form of neurodegenerative dementia in the United States. According to Alzheimer’s disease facts and figures in 2023, there are about 6.7 million people suffering from dementia in the U.S., and this number is projected to grow to 13.8 million by 2060.
One leading theory of the progression of AD posits that the disease is caused by the overaccumulation of a protein called amyloid-beta (Aβ), which naturally exists in the brain. Normally, Aβ is cleaved off by proteins and gets cleared away by the brain's cleaning system. However, sometimes these fragments can start sticking together. When too many Aβ pieces clump together, they form plaques between brain cells.
Now you might be wondering: how does overaccumulation lead to AD? Well, it turns out that Aβ is neurotoxic, meaning that it could cause disruption and even death of neural cells [5]. Researchers at Massachusetts General Hospital and collaborating institutions investigated Aβ neurotoxicity by injecting synthetic Aβ into rat cerebral cortex. After 7 days, they observed significant neuronal loss at the injection site and neuritic degeneration in surrounding neurons. Neuritic refers to something that relates to or affects the branch-like projections that extend from the neurons. These findings suggest that Aβ may contribute to neurodegeneration in AD.
However, the role of Aβ in the progression of AD remains controversial. Some argue that Aβ accumulation alone is insufficient to cause cognitive decline, pointing to individuals with significant Aβ buildup who maintain normal cognitive function [6]. Additionally, therapies targeting Aβ have thus far shown limited success in clinical trials.
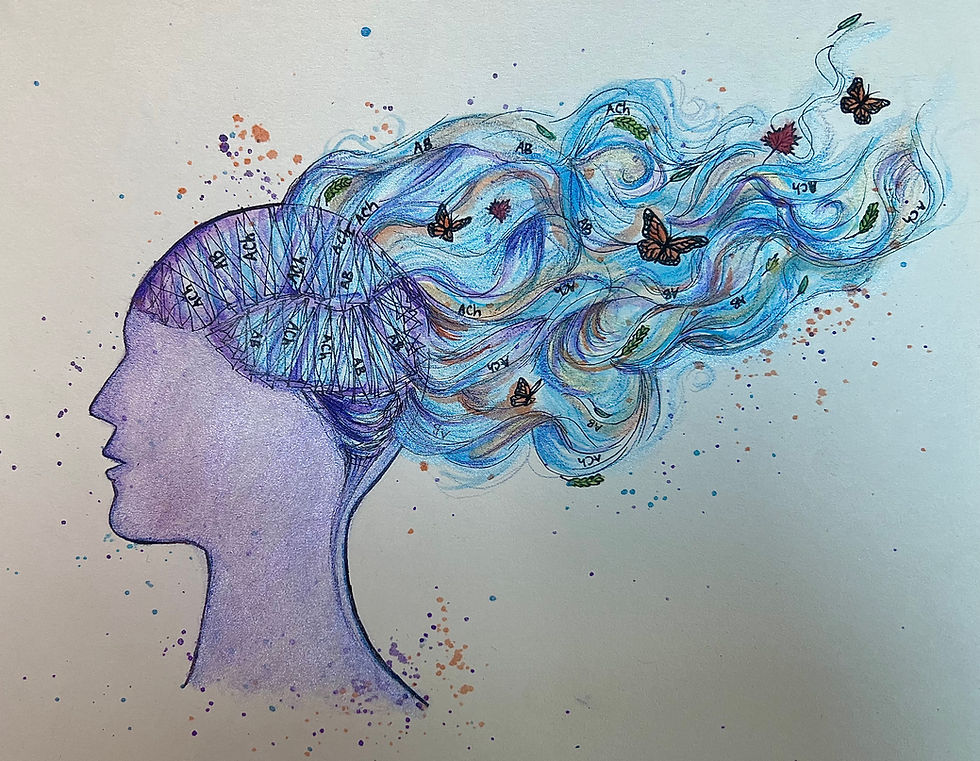
Other mechanisms are also implicated in Alzheimer's pathology. For example, Tau proteins are tiny support structures that help maintain pathways inside brain cells. When these Tau proteins don't work properly, they start to clump together and twist abnormally. This messes up the transport pathways inside brain cells, making it impossible for important materials to move around–-kind of like a blocked road. Without these materials getting where they need to go, the brain cells eventually die [7]. Also, the brain's memory and learning system (called the cholinergic system) starts breaking down, which makes it harder to learn and remember things [8, 9]. While these complex brain changes have long challenged researchers, artificial intelligence is emerging as a powerful tool that could revolutionize how we understand and treat Alzheimer's Disease.
The Promise of Artificial Intelligence in Alzheimer's Research
Artificial intelligence, particularly machine learning, has already revolutionized how digital data is analyzed, and will no doubt be used in Alzheimer’s research [2]. Instead of researchers having to manually sort through thousands of medical records, brain scans, or test results, AI can quickly analyze large amounts of information and find hidden patterns that humans might miss. Machine learning is a type of artificial intelligence where computers learn from experience-–much like humans do—without being specifically programmed for each task. One promising application is in early detection and diagnosis [10]. Since pathological changes begin decades before clinical symptoms appear, AI models could help identify Alzheimer's in its presymptomatic stage by recognizing subtle patterns in brain scans, blood biomarkers, and cognitive assessments. A biomarker is a measurable signal in the body that indicates a specific health condition, like a warning light on a car's dashboard. This capability serves two crucial purposes: enabling earlier intervention when treatments may be more effective, and helping researchers understand how AD pathology develops and progresses before causing cognitive decline. Early detection thus becomes vital not only for patient care but also for uncovering the fundamental mechanisms of disease onset.
Deep learning, a subset of machine learning that teaches computers to learn in layers like the human brain does, is particularly well-suited for analyzing medical imaging data [11]. Convolutional neural networks (CNNs) can track changes in brain structure over time, similar to how a time-lapse camera captures a plant's growth. In Alzheimer's disease, certain brain regions gradually shrink, particularly the hippocampus, a crucial area for memory. CNNs can identify the hippocampus in brain MRI scans and measure its size precisely, helping doctors detect and monitor this shrinkage, which happens before memory problems become noticeable. This automation could enhance the efficiency and consistency of image analysis in clinical settings and research. However, there are still challenges to overcome. AI systems need huge amounts of patient data to learn effectively—like needing thousands of brain scans and medical records to recognize patterns accurately. Another concern is understanding how AI makes its decisions—doctors need to know why the AI suggests certain conclusions. There's also worry about fairness, for if the AI system is trained mostly

on data from certain groups of people (like one particular age group or ethnic background), it may not translate to a broad patient population. Solving these problems is important as we continue to use AI more in Alzheimer's research and treatment [12].
AI in biomarker discovery and validation
The application of AI in biomarker discovery and validation represents a significant leap forward in our ability to detect and monitor AD. It could be something in your blood, a change in brain structure visible on a scan, or a specific protein level that tells doctors whether a disease might be present or how it's progressing.
Machine learning algorithms, particularly deep learning models, have demonstrated remarkable capabilities in identifying novel biomarkers for AD [13]. These AI systems can analyze vast and complex datasets to detect patterns and correlations in people with AD that human researchers might otherwise miss. For instance, a study by Whelan et al. used machine learning to analyze proteins from cerebrospinal fluid (CSF) and blood plasma samples. CSF is like a watery cushion in your brain and spinal cord that provides protection and nutrients to brain cells. While CSF offers valuable information about brain health, collecting it requires an invasive procedure where a needle must be inserted into the lower spine. In the study, the AI model was able to identify a panel of protein biomarkers that could distinguish AD patients from people without AD with high accuracy, highlighting the presence of possible diagnostic markers and such models' ability to identify them. Notably, some of these biomarkers were also present in blood plasma, suggesting the potential for less invasive and more cost-effective diagnostic methods. A breakthrough study by Nakamura et al. used machine learning to develop a blood test that can predict the accumulation of amyloid in the brain with 90% accuracy. This kind of progress could make early screening for AD much more accessible and widespread.
AI's capacity for analyzing longitudinal data, which is information collected from the same source over a long period of time, is particularly valuable in understanding the progression of AD. Machine learning models can track changes in biomarker levels over time, potentially identifying early indicators of disease onset or progression long before clinical symptoms appear. For example, an AI system developed by Oxtoby et al. analyzed thousands of patient records from the Alzheimer's Disease Neuroimaging Initiative (ADNI) to map how AD unfolds over time [14]. By studying brain scans, spinal fluid tests, and cognitive assessments, the AI revealed the sequence of changes, from early protein buildup to later brain tissue loss and memory problems. This timeline helps researchers target treatments and doctors predict disease progression in patients.
By analyzing an individual's biomarker profile in the context of large-scale population data, AI models can provide personalized risk assessments for AD. This approach, often referred to as precision medicine, could enable earlier interventions and more targeted treatment strategies. A study by Khanna et al. demonstrated this potential by developing a machine learning model that combined genetic, clinical, and biomarker data to predict an individual's risk of developing AD [15]. The model outperformed traditional risk assessment tools like simple brain volume measurements and could potentially identify high-risk individuals decades before symptom onset.
This kind of personalized risk assessment could be transformative in AD care. It could allow for targeted interventions in individuals long before symptoms appear, potentially delaying or even preventing the onset of the disease. Moreover, it could help guide decisions about participation in clinical trials, ensuring that new treatments are tested on the individuals most likely to benefit from them.
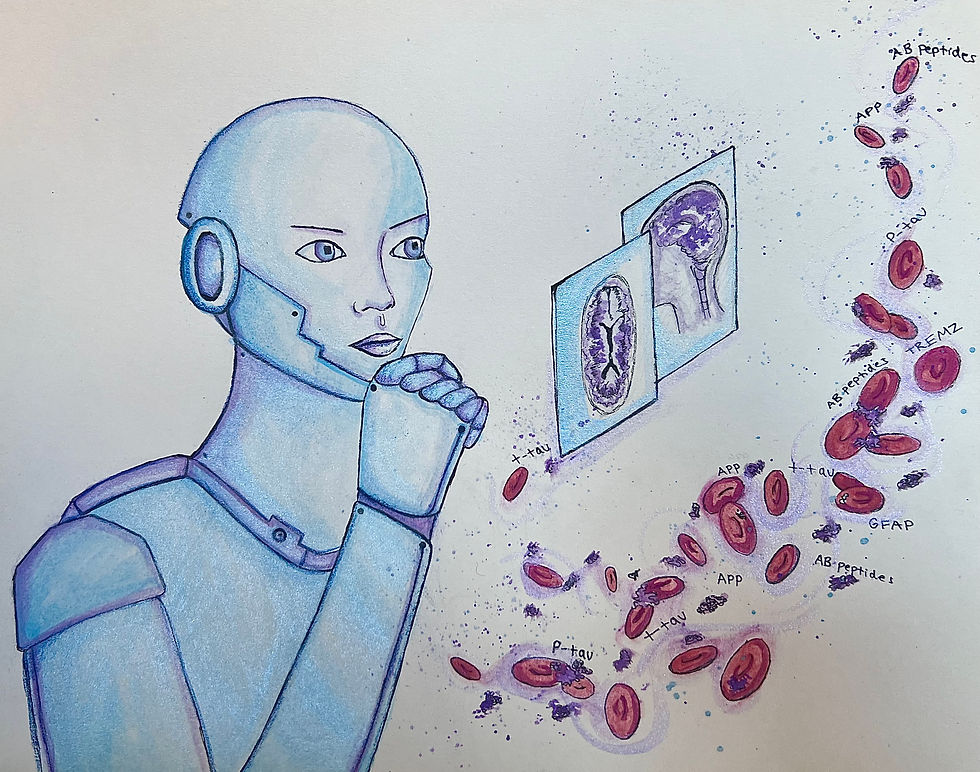
While not directly related to biomarker detection, it's worth noting that AI is also playing a significant role in drug discovery and development for AD. AI is also transforming drug discovery—machine learning can rapidly search through millions of potential drug compounds, dramatically speeding up the traditional process of finding new treatments. Machine learning models can screen vast libraries of compounds to identify potential therapeutic candidates more rapidly and cost-effectively than traditional methods. For example, a study by Zhang et al. used a deep learning model to screen a library of over 30 million small molecules, identifying several promising candidates for AD treatment [16]. This AI-driven approach to drug discovery could potentially accelerate the development of new treatments for AD, bringing hope to patients and families waiting for breakthroughs.

Challenges and Future Directions
However, for AI to reach its full potential in both drug discovery and biomarker detection, researchers must first overcome significant challenges in how data is collected and processed.
A. Data Quality and Standardization
Imagine you're trying to build a massive puzzle, but some pieces are missing, others are blurry, and some might even be from different puzzles altogether. That's similar to the challenge researchers face when working with AI in Alzheimer's research. They need lots of clear, accurate information from many different patients to train AI to make reliable predictions.
If you want to finish the puzzle, you have to try many ways of putting two puzzle pieces together. If you want the recipes to work for everyone, you need to test them with different ingredients and cooking methods. Similarly, researchers need information from many different types of patients to make sure their AI tools work well for everyone, not just certain groups of people.
There are some big projects, like ADNI and GAAIN, where researchers pool different types of patient information - from brain scans and blood tests to genetic data and cognitive test scores. It's like how weather forecasters from different regions share their data to make better predictions. By working together, researchers can build better AI tools to fight Alzheimer's.
But here's the tricky part: this information is very personal, so researchers need to protect people's private information while still being able to use it for their studies. Developing robust frameworks for protecting patient data while enabling research access is an ongoing challenge. Researchers and policymakers are working to strike a balance between data accessibility for scientific advancement and stringent protections for patient privacy, a critical consideration as AI continues to play a larger role in Alzheimer's research and care.
B. Interpretability and Transparency
The "black box" nature of many AI algorithms poses significant challenges in healthcare applications [17], particularly in the sensitive area of Alzheimer's research and diagnosis. What is “black box” nature then? It’s the uncertainty of the decision-making process of algorithms that makes it untrustworthy to researchers and medical professionals since we often can’t fully explain the paths AI uses to draw conclusions.
This is especially important when we're dealing with something as serious as Alzheimer's disease. Imagine if your doctor told you they were making an important decision about your health but couldn't explain why—that wouldn't feel right, would it? That's why researchers are working hard to create AI that can "show its work," just like your math teacher asks you to.
Collaboration between AI researchers and clinicians is essential to bridge the gap between technical capabilities and clinical needs, ensuring that AI tools are both powerful and practical for real-world use. This interdisciplinary approach is crucial for developing AI systems that not only perform well in laboratory settings but also integrate seamlessly into clinical workflows and meet the real-world needs of Alzheimer's patients and healthcare providers.
Conclusion
As we've seen, artificial intelligence is transforming how we tackle three crucial problems in Alzheimer's research: finding reliable biomarkers, catching the disease early, and developing new drugs. AI's ability to spot hidden patterns in complex data has already led to promising breakthroughs, like blood tests that can predict amyloid buildup with remarkable accuracy and computer models that can sift through millions of potential drug compounds.
These advances matter because traditional research methods have struggled with Alzheimer's complex nature. By analyzing long-term data from patients, AI tools are helping us map out how the disease unfolds over time. This understanding is vital since brain changes begin decades before memory problems appear - and the earlier we can intervene, the better our chances of treating patients.
Yet we face real challenges in putting these AI solutions to work. We need better ways to standardize data from different research centers, and doctors need to understand how AI makes its decisions before they can trust it in clinical practice. Patient privacy and data security can't be compromised as we build these systems.
The stakes are high-–Alzheimer's affects 6.7 million Americans today, and that number could more than double by 2060. But for the first time, AI is giving us practical tools to detect the disease earlier and develop more targeted treatments. While we still have work to do, these advances offer real hope for tackling this devastating illness more effectively than ever before.
REFERENCES:
1. Briggs, R., Kennelly, S. P., & O’Neill, D. (2016). Drug treatments in Alzheimer’s disease. Clinical Medicine, 16(3), 247–253. https://doi.org/10.7861/clinmedicine.16-3-247
2. Fabrizio, C., Termine, A., Caltagirone, C., & Sancesario, G. (2021). Artificial Intelligence for Alzheimer’s Disease: Promise or Challenge? Diagnostics, 11(8), 1473. https://doi.org/10.3390/diagnostics11081473
3. Lane, C. A., Hardy, J., & Schott, J. M. (2018). Alzheimer’s disease. European Journal of Neurology, 25(1), 59–70. https://doi.org/10.1111/ene.13439
4. Soria Lopez, J. A., González, H. M., & Léger, G. C. (2019). Alzheimer’s disease. In Handbook of Clinical Neurology (Vol. 167, pp. 231–255). Elsevier. https://doi.org/10.1016/B978-0-12-804766-8.00013-3
5. Reiss, A. B., Arain, H. A., Stecker, M. M., Siegart, N. M., & Kasselman, L. J. (2018). Amyloid toxicity in Alzheimer’s disease. Reviews in the Neurosciences, 29(6), 613–627. https://doi.org/10.1515/revneuro-2017-0063
6. Jonsson, T., Atwal, J. K., Steinberg, S., Snaedal, J., Jonsson, P. V., Bjornsson, S., … Stefansson, K. (2012). A mutation in APP protects against Alzheimer’s disease and age-related cognitive decline. Nature, 488(7409), 96–99. https://doi.org/10.1038/nature11283
7. Jouanne, M., Rault, S., & Voisin-Chiret, A.-S. (2017). Tau protein aggregation in Alzheimer’s disease: An attractive target for the development of novel therapeutic agents. European Journal of Medicinal Chemistry, 139, 153–167. https://doi.org/10.1016/j.ejmech.2017.07.070
8. H. Ferreira-Vieira, T., M. Guimaraes, I., R. Silva, F., & M. Ribeiro, F. (2016). Alzheimer’s disease: Targeting the Cholinergic System. Current Neuropharmacology, 14(1), 101–115. https://doi.org/10.2174/1570159X13666150716165726
9. Grossberg, S. (2017). Acetylcholine Neuromodulation in Normal and Abnormal Learning and Memory: Vigilance Control in Waking, Sleep, Autism, Amnesia and Alzheimer’s Disease. Frontiers in Neural Circuits, 11, 82. https://doi.org/10.3389/fncir.2017.00082
10. Ding, Y., Sohn, J. H., Kawczynski, M. G., Trivedi, H., Harnish, R., Jenkins, N. W., … Franc, B. L. (2019). A Deep Learning Model to Predict a Diagnosis of Alzheimer Disease by Using 18 F-FDG PET of the Brain. Radiology, 290(2), 456–464. https://doi.org/10.1148/radiol.2018180958
11. Jo, T., Nho, K., & Saykin, A. J. (2019). Deep Learning in Alzheimer’s Disease: Diagnostic Classification and Prognostic Prediction Using Neuroimaging Data. Frontiers in Aging Neuroscience, 11, 220. https://doi.org/10.3389/fnagi.2019.00220
12. Gianfrancesco, M. A., Tamang, S., Yazdany, J., & Schmajuk, G. (2018). Potential Biases in Machine Learning Algorithms Using Electronic Health Record Data. JAMA internal medicine, 178(11), 1544–1547. https://doi.org/10.1001/jamainternmed.2018.3763
13. Whelan, C. D., Mattsson, N., Nagle, M. W., Vijayaraghavan, S., Hyde, C., Janelidze, S., … Hansson, O. (2019). Multiplex proteomics identifies novel CSF and plasma biomarkers of early Alzheimer’s disease. Acta Neuropathologica Communications, 7(1), 169. https://doi.org/10.1186/s40478-019-0795-2
14. Oxtoby, N. P., Shand, C., Cash, D. M., Alexander, D. C., & Barkhof, F. (2022). Targeted Screening for Alzheimer’s Disease Clinical Trials Using Data-Driven Disease Progression Models. Frontiers in Artificial Intelligence, 5, 660581. https://doi.org/10.3389/frai.2022.660581
15. Khanna, S., Domingo-Fernández, D., Iyappan, A., Emon, M. A., Hofmann-Apitius, M., & Fröhlich, H. (2018). Using Multi-Scale Genetic, Neuroimaging and Clinical Data for Predicting Alzheimer’s Disease and Reconstruction of Relevant Biological Mechanisms. Scientific Reports, 8(1), 11173. https://doi.org/10.1038/s41598-018-29433-3
16. Zhang, F., Pan, B., Shao, P., Liu, P., Shen, S., Yao, P., & Xu, R. X. (2022). A Single Model Deep Learning Approach for Alzheimer’s Disease Diagnosis. Neuroscience, 491, 200–214. https://doi.org/10.1016/j.neuroscience.2022.03.026
17. Rudin, C. (2019, September 22). Stop Explaining Black Box Machine Learning Models for High Stakes Decisions and Use Interpretable Models Instead. arXiv. https://doi.org/10.48550/arXiv.1811.10154
Commentaires