by Stella Dong
art by Stephen Suh
The Diagnostic and Statistical Manual of Mental Disorders defines a mental disorder as a syndrome that shows a significant disturbance in an individual’s emotion, cognition, or behavior that reflects a harmful dysfunction in one’s psychological or social life [1].
Given that 22.8% of Americans reported having a mental illness or disorder in a 2021 survey, understanding what constitutes mental disorders and how they are diagnosed and treated is vital [2]. Unlike physical diseases such as cancer or diabetes, it is difficult to diagnose mental disorders through precise examinations. Currently, diagnosing mental disorders relies mostly on the Diagnostic and Statistical Manual of Mental Disorders (DSM) published by the American Psychiatric Association (APA) [3]. The manual includes 157 mental disorders identified by the APA and lists criteria including symptoms and feelings over a period of time that a person must meet in order to be officially diagnosed with a mental illness [1]. However, these rather subjective criteria, which often depend on an individual’s self-report, seem to be lacking a “quantitative” component. For example, two of the diagnostic criteria for major depressive disorder (MDD) include: a consistent presence of depressed mood and diminished interest or pleasure in nearly all activities, both of which can be indicated as observed by others or by self-report [1].
A major obstacle within the study of psychiatric disorders is our limited understanding of the brain functions associated with our behaviors [4]. We know well that our brain is composed of specialized cells, or neurons, that transmit information to neighboring neurons. But how do these clusters of neurons create feelings, thoughts, and emotions? We know that there must be an assembly of neurons at work, but what are we really observing when we see these neuronal activities? How can we use the information to interpret our behavior, or make predictions about it? This we have no answer for.
For example, there are networks in the brain responsible for processing visual information [4]. Our eyes take in visual stimuli from the world and convert it into electrical signals to be processed by the brain. Each part of the visual processing regions of the brain extracts one element from the things we see (shape, color, movement). Then somehow, we are able to put all these pieces of information together to see one object with a coherent experience [4]. The difficulty to understand the connections between the biological brain circuitry and higher cognitive functions further challenges us to fully understand the neurobiology that accounts for mental disorders [5]. Therefore, mental disorders cannot yet be diagnosed with a simple brain scan.
However, the recent development of computational psychiatry brings together cross-disciplinary subjects to model behaviors and their underlying neural networks in psychiatric patients, using data-driven and theory-driven approaches [6]. Computational psychiatry is an interdisciplinary field that combines theories from computer science, mathematics, neuroscience, and psychiatry to develop mathematical models and computational tools for understanding the neural and cognitive processes underlying mental disorders, as well as for predicting and treating these disorders [7]. It aims to leverage the power of computation and quantitative methods to gain a better understanding of the brain and behavior, and to develop new approaches to diagnosis, treatment, and prevention of mental illnesses [7].
The History of Psychiatric Disorders
The notion of mental illness was present long before systematic diagnoses and categorizations of disorders were created. To better understand psychiatric disorders, we must look at the perception of mental illness across history. Dating as early as 6500 BC, prehistoric bones and cave art identify surgical drilling of holes in skulls to treat epilepsy and release evil spirits trapped within the skull [8]. Around 400 BC, Hippocrates distinguished mental illness from superstition and religion by introducing the belief that mental illness originates from an excess of one of the four essential bodily fluids. Fast forward to the Middle Ages, religion played an important role in explaining the phenomenon of mental illness, deeming it a result of demonic possession. The idea that the mentally-ill are “crazy” also played a role in the lack of proper treatment methods. Starting in the 16th century, doctors started to practice medical treatments for people with mental health issues, such as surgeries that create a hole in one’s skull [8]. The rise of mental hospitals also allowed more organized treatment for mental illness [9]. The “insane asylums” in the early 1900s were institutions in which little therapeutic activity actually occurred [9]. In the first half of the 20th century, psychoanalysis took over the psychiatric studies—combining behavioral, cognitive, cognitive-behavioral, psychodynamic, and client-centered approaches with psychotherapy [10].
In 1952, the APA published the first DSM, a single shared systematic categorization of mental disorders and diagnoses among psychiatric clinicians [1]. From then on, the DSM went through various revisions to improve its classification system [8]. It provided a necessary shared language for clinicians and created a financial tie between some DSM panel members and the pharmaceutical industry, where revision of the diagnostic criteria can be influenced by the for-profit entities that manufacture drugs used in the treatment of mental illness [8, 10].
Treatments For Psychiatric Disorders
Currently, the major criteria used for diagnosing mental disorders is based on the DSM-5, in addition to some possible physical examinations to eliminate other possible medical conditions that can contribute to the abnormal symptom [11]. A treatment plan is then created based on the diagnosis [11]. The treatment plans can be categorized as either somatic or psychotherapeutic [12]. Somatic treatments include medication, and other therapies such as the electroconvulsive therapy to cause changes in the brain chemistry using electric currents [12, 13]. This method is found to sometimes be able to quickly reverse symptoms of certain mental health conditions [13]. On the other hand, psychotherapeutic treatments include psychotherapy, behavioral therapy techniques, and hypnotherapy [12]. Usually, a treatment that involves both categories is more efficient than either used alone [12].
While the pre-eminent role of diagnosis in medicine is to determine the exact nature of a patient’s disease in order to administer the optimal treatment, little discussion of this aspect can be found in research studies around DSM-5, despite its effort in updating the definition and diagnostic criteria of different mental disorders [14]. This results in the current situation where effective treatment exists but is not precise. For example, treatments for major classes of disorders such as depression, anxiety disorders, schizophrenia, and bipolar disorders are available, and effective for a large number of patients. However, some treatments such as antidepressant medications affect a broad class of disorders: various anxiety, mood, and other disorders [14]. Similarly, behavioral treatments such as cognitive-behavioral therapy—established initially to treat depression—have now expanded to treat virtually all mental illnesses [15].
RDoC Framework
To help develop research that can better organize and connect neurological and behavioral measures in mental illness, the National Institute of Mental Health (NIMH) established the Research Criteria (RDoC) project [16]. Rather than viewing mental illnesses as lists of symptoms based on behavioral descriptions, the system created six major domains of basic human neurobehavioral functioning: Positive Valence, Negative Valence, Social Processes, Cognitive, Sensorimotor, and Arousal/Regulatory. Each domain is then broken down into different aspects of psychological and biological dimensions, which are studied in both normal and abnormal functioning [16]. This shift toward behavioral and neural functioning in psychopathology created novel approaches among research studies. For example, enhanced assessment of behavioral and cognitive functions enables quantitative measurements to foster computational techniques in comparison to qualitative symptom
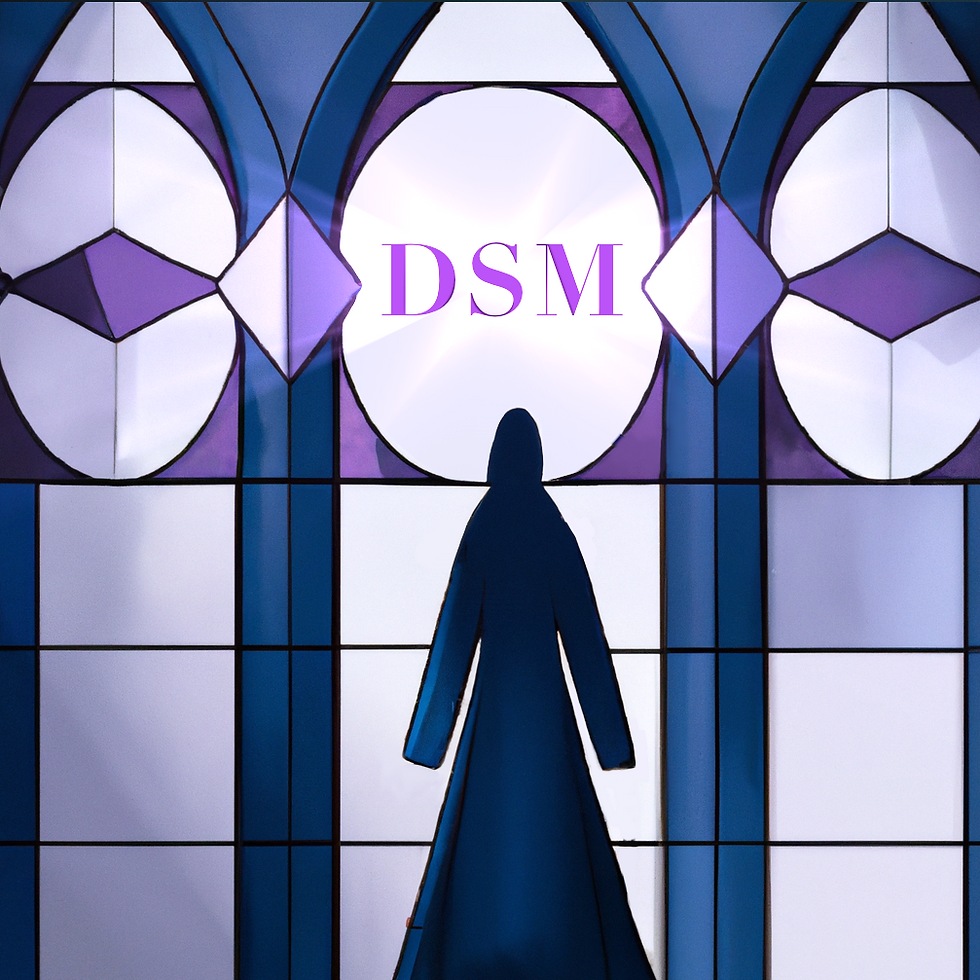
reports [17]. The functional domain approach enables researchers to explore mechanisms that are shared by multiple mental disorders [17]. The development of such a framework creates the hope for developing a precision medicine approach for mental illnesses [14]. With this high-dimensional approach to the organization of mental illness, computational psychiatry can be particularly helpful in finding how the domains in RDoC are connected with each other, and bridge recent findings in neurobiological changes in the brain and psychopathology [18].
So, what is computational psychiatry?
Computational psychiatry aims to describe the relationship between the neurobiology of the brain, its environment, and mental symptoms in computational terms [18]. It builds models representing the ways in which the brain functions in relation to thoughts and behaviors. Through establishing mathematical relationships between symptoms, environments, and neurobiology, it hopes to provide tools to identify causes of particular symptoms in individual patients, and aids in improving corresponding treatments. By applying the model to patients with mental illnesses, changes in the parameters of such mathematical models reflect the cause of changes in certain behaviors among certain patients [18].
Currently, the majority of research in computational psychiatry has been focusing on the investigation of learning and decision-making, which are the core components of cognitive functions [19]. Of the many computational psychiatry models established, the reinforcement learning (RL) model is widely used in recent studies and has found widespread application to empirical data. Since many psychiatric disorders are characterized by suffering and the inability to change one’s behavior regardless of negative consequences, understanding how one takes actions in receiving reward is important to gain insight into its pathological alterations [19].
As classical and instrumental conditioning make up the most basic paradigms for decision-making, reinforcement learning models can be viewed as a computational framework for conditioning that provides a further understanding of the conditioned behavior [20]. RL models generate predictions for optimal behavior, suggesting how predictions and behavioral adjustments can be achieved for optimal outcomes, and explicitly show the parameters needed to be adjusted to achieve such an outcome [19, 20]. In the RL model, an individual interacts with their environment, takes action according to the environment, and observes some outcome. Through changes in states of the environment and probabilities of outcomes, an individual can adapt to make better decisions about their future actions. Usually, action changes with the goal of maximizing a desired outcome and avoiding an undesirable outcome [19, 20]. The most simple form of such model is called a Q-learning model:
Q(A)t+1 Q(A)t+ [Rt-Q(A)t]
The equation shows how the value of future action is produced [21]. Here, it is the sum of the value of the current action, denoted as Q(A)t, and a constantly updating factor based on one’s expectation of the future outcome, denoted as [Rt-Q(A)t]. It includes the prediction error (PRE), [Rt-Q(A)t], which is the difference between the actual reward received of the current trial (Rt) and the expectation of a reward of the trial (Q(A)t). A larger difference
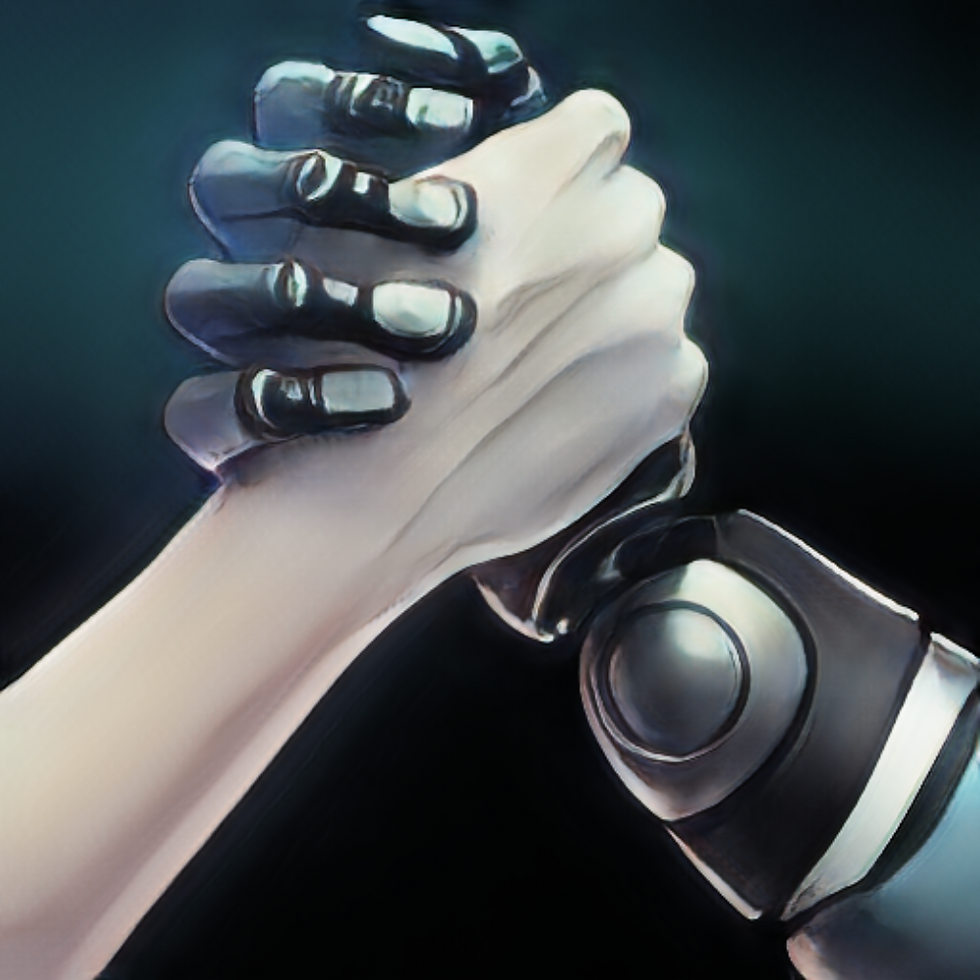
between the expectation and the reward results in a larger PRE value. This value is further adjusted by , the learning rate parameter. This parameter ranges from zero to one and indicates the rate at which an individual updates its prediction error in attribution to the future value of action. A higher learning rate indicates a higher influence of the PRE in adjusting the future value of action [21].
Emerging evidence suggests that changes in the way rewards and punishments are learned may contribute to symptoms of deficit in decision-making behaviors in major depressive disorder (MDD) [19]. These behavioral differences are shown to be correlated with altered brain activity in various regions responsive to rewarding and punishing feedback. Using the Q learning model, however, we can examine the interaction between dynamic brain activity and its relation to behavior among people with depression, further understanding how the various brain regions and their interactions are functionally and structurally altered in depression. The role of reward in reinforcement learning has been studied in the context of RL models of dopaminergic activity, such as ways in which dopaminergic neurons encode an expected and experienced outcome differently. It can also pair behavioral decision-making tasks with fMRI to examine changes in choice-making actions and correlations in neural response through learning from rewards and punishments [19].
A study by Huys et al. distinguished the effects of anhedonia (an inability to experience pleasure or diminished reactivity to pleasurable stimuli) and dopamine in terms of changes in sensitivity to rewards and changes in PRE updates [22]. Anhedonia is one of the diagnostic criteria listed in DSM-5 for MDD. This can be observed when participants were less impacted by the reward when it occurred [22]. This reduction in “liking” has been found to be associated with μ-opioid signaling in the nucleus accumbens region of the brain, where studies have found prefrontal changes in μ-opioid receptors in MDD [22, 23]. μ-opioids are involved with generating pleasurable sensations when receiving rewards, and simulations of their receptors in the nucleus accumbens increases feelings of pleasure [24]. However, the RL model suggests that manipulation of dopamine levels is shown in changes in the learning rate [22]. This is supported by studies confirming that dopamine agonists, medications used to increase dopamine levels, can boost prediction errors by restoring the learning rate [25]. Therefore, alterations in dopamine levels impact the expected value rather than the value of the reward itself, suggesting that dopamine is not correlated with anhedonia [22]. Using the RL model, research studies distinguished the effect of dopamine on reward-seeking behavior. Particularly, dopamine mediates the “wanting”—the learning rate of prediction errors—rather than the “liking”, or the sensitivity to the value of reward itself. This implies that treating anhedonia in depressive states by manipulating dopamine levels may not result in the exact desired behavioral changes, suggesting more investigations need to be done to better distinguish the behavioral consequences resulting from alterations in dopamine and in reward sensitivity [22].
Other than providing insight into the causation of depression, studies in computational psychiatry can also suggest new directions for treatments that better fit each individual. Cognitive behavioral therapy (CBT) is a type of talk therapy that is led by a therapist to identify and rationalize negative thoughts in order to replace them with healthier ones [26]. It sometimes works as well as antidepressant drugs for some types of depression [26]. A central goal of CBT is to support patients in moving toward their goals, which typically involve helping patients to adapt their beliefs and behavior to their current environment [27]. In computational modeling, this means the alteration of mapping between states the person is in and their responding actions. For example, imagine a scenario where a dancer with depression reports that they now get less pleasure (or reward) from an old activity they used to enjoy, such as going to dance practices, and have stopped going completely. A possible explanation is that there is a lack of positive reinforcement from attending the dance practice, or a negative reinforcement of avoiding experiencing negative emotions if they do not perform perfectly in the practice. Hence, the value of going to dance practice reduces, and further reduces future expectations of such an action as its expected value has decreased. Successful therapy would attempt to reverse this change. Therefore, parameters from the RL model may be useful to measure the effects of CBT. For example, a way to track improvement in therapy can be done by evaluating a decrease in the tendency to assign negative value to novel stimuli, which may reflect a reduction in over-generalizing prior negative knowledge, and increased flexibility to update future values [28]. By tracking individual patients’ progress using computational models, one can adjust treatment plans accordingly and determine when improvements have reached an asymptote [28].
Computational Psychiatry as the Rising Star?
As discussed in the above sections, computational psychiatry seems to be an exciting and promising field to help us better understand the connections between the neurological and the behavioral basis that leads to psychiatric disorders. If the computational approach does turn out to be effective, then one can link precise elements of the models created based on large-scale datasets to measurable aspects of behavior and to molecular and neural substrates that can be independently measured [29]. Moreover, the model can distinguish dysfunctions that are related to but different from the existing categories of criteria. However, the field also faces some challenges. Many concepts can be hard to put in mathematically precise terms. Feelings and behaviors that come natural to humans may in fact be very complex in computational representations, and we are still very far from being able to fully grasp both the concepts and the mathematical tools required to realize these concepts. Therefore, it will be interesting to see where—and how far—the field can take us in our understanding of mental illness.
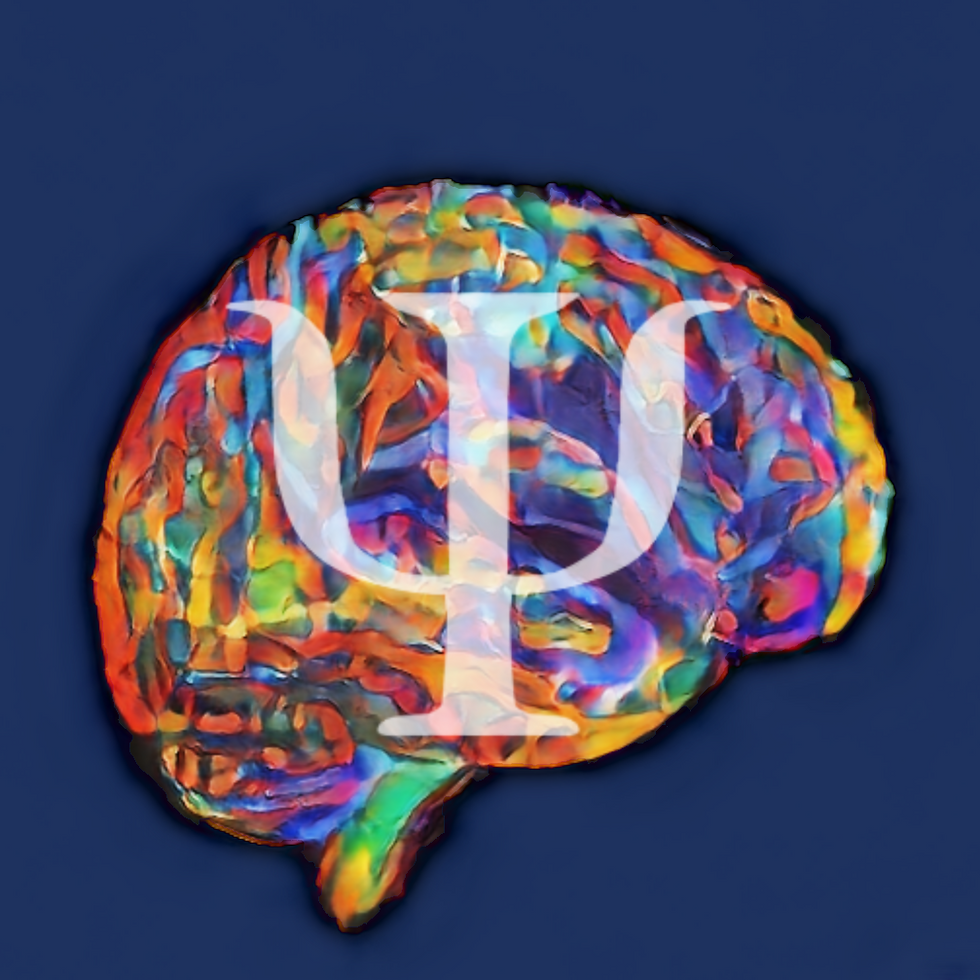
REFERENCES
American Psychiatric Association, & American Psychiatric Association (Eds.). (2013). Diagnostic and statistical manual of mental disorders: DSM-5 (5th ed.). Washington, D.C: American Psychiatric Association.
Substance Abuse and Mental Health Services Administration. (2022). Key substance use and mental health indicators in the United States: Results from the 2021 National Survey on Drug Use and Health (HHS Publication No. PEP22-07-01-005, NSDUH Series H-57). Center for Behavioral Health Statistics and Quality, Substance Abuse and Mental Health Services Administration. https://www.samhsa.gov/data/report/2021-nsduh-annual-national-report
Understanding Your Diagnosis. (n.d.). NAMI | National Alliance on Mental Illness. Retrieved February 13, 2023, from https://www.nami.org/Your-Journey/Individuals-with-Mental-Illness/Understanding-Your-Diagnosis
Herbert, J. (2015, October 9). Cracking the skull open.
Hyman, S. E. (2007). Can neuroscience be integrated into the DSM-V? Nature Reviews Neuroscience, 8(9), 725–732. https://doi.org/10.1038/nrn2218
Huys, Q. J. M., Maia, T. V., & Frank, M. J. (2016). Computational psychiatry as a bridge from neuroscience to clinical applications. Nature Neuroscience, 19(3), 404–413. https://doi.org/10.1038/nn.4238
Computational psychiatry. neurocpu. (n.d.). Retrieved March 28, 2023, from https://www.neurocpu.org/computational-psychiatry
Farreras, I. G. (2023). History of mental illness. In R. Biswas-Diener & E. Diener (Eds), Noba textbook series: Psychology. Champaign, IL: DEF publishers. Retrieved from http://noba.to/65w3s7ex
Crossley, N. (2006). Contesting Psychiatry: Social Movements in Mental Health. Psychology Press.
Cosgrove, L., Krimsky, S., Vijayaraghavan, M., & Schneider, L. (2006). Financial ties between DSM-IV panel members and the pharmaceutical industry. Psychotherapy and Psychosomatics, 75(3), 154–160. https://doi.org/10.1159/000091772
Balogh, E. P., Miller, B. T., Ball, J. R., Care, C. on D. E. in H., Services, B. on H. C., Medicine, I. of, & The National Academies of Sciences, E. (2015). The Diagnostic Process. In Improving Diagnosis in Health Care. National Academies Press (US). https://www.ncbi.nlm.nih.gov/books/NBK338593/
First, M. B. (2020, April). Treatment of Mental Illness - Mental Health Disorders. Merck Manuals Consumer Version. Retrieved February 21, 2023, from https://www.merckmanuals.com/home/mental-health-disorders/overview-of-mental-health-care/treatment-of-mental-illness
Electroconvulsive therapy (ECT)—Mayo Clinic. (n.d.). Retrieved March 23, 2023, from https://www.mayoclinic.org/tests-procedures/electroconvulsive-therapy/about/pac-20393894
Cuthbert, B. N., & Insel, T. R. (2013). Toward the future of psychiatric diagnosis: The seven pillars of RDoC. BMC Medicine, 11(1), 126. https://doi.org/10.1186/1741-7015-11-126
Sensky, T., Turkington, D., Kingdon, D., Scott, J. L., Scott, J., Siddle, R., … Barnes, T. R. (2000). A randomized controlled trial of cognitive-behavioral therapy for persistent symptoms in schizophrenia resistant to medication. Archives of General Psychiatry, 57(2), 165–172. https://doi.org/10.1001/archpsyc.57.2.165
About RDoC. (n.d.). National Institute of Mental Health (NIMH). Retrieved April 14, 2023, from https://www.nimh.nih.gov/research/research-funded-by-nimh/rdoc/about-rdoc
Cuthbert, B. N. (2020). The role of RDoC in future classification of mental disorders. Dialogues in Clinical Neuroscience, 22(1), 81–85. https://doi.org/10.31887/DCNS.2020.22.1/bcuthbert
Adams, R. A., Huys, Q. J. M., & Roiser, J. P. (2016). Computational Psychiatry: towards a mathematically informed understanding of mental illness. Journal of Neurology, Neurosurgery, and Psychiatry, 87(1), 53–63. https://doi.org/10.1136/jnnp-2015-310737
Liebenow, B., Jones, R., DiMarco, E., Trattner, J. D., Humphries, J., Sands, L. P., … Kishida, K. T. (2022). Computational reinforcement learning, reward (and punishment), and dopamine in psychiatric disorders. Frontiers in Psychiatry, 13. Retrieved from https://www.frontiersin.org/articles/10.3389/fpsyt.2022.886297
Niv, Y. (2009). Reinforcement learning in the brain. Journal of Mathematical Psychology, 53(3), 139–154. https://doi.org/10.1016/j.jmp.2008.12.005
Daw, N. D., & Tobler, P. N. (2014). Value Learning through Reinforcement. In Neuroeconomics (pp. 283–298). Elsevier. https://doi.org/10.1016/B978-0-12-416008-8.00015-2
Huys, Q. J., Pizzagalli, D. A., Bogdan, R., & Dayan, P. (2013). Mapping anhedonia onto reinforcement learning: A behavioural meta-analysis. Biology of Mood & Anxiety Disorders, 3(1), 12. https://doi.org/10.1186/2045-5380-3-12
Kennedy, S. E., Koeppe, R. A., Young, E. A., & Zubieta, J.-K. (2006). Dysregulation of Endogenous Opioid Emotion Regulation Circuitry in Major Depression in Women. Archives of General Psychiatry, 63(11), 1199–1208. https://doi.org/10.1001/archpsyc.63.11.1199
Nummenmaa, L., Saanijoki, T., Tuominen, L., Hirvonen, J., Tuulari, J. J., Nuutila, P., & Kalliokoski, K. (2018). μ-opioid receptor system mediates reward processing in humans. Nature Communications, 9(1), 1500. https://doi.org/10.1038/s41467-018-03848-y
Chowdhury, R., Guitart-Masip, M., Lambert, C., Dayan, P., Huys, Q., Düzel, E., & Dolan, R. J. (2013). Dopamine restores reward prediction errors in old age. Nature Neuroscience, 16(5), 648–653. https://doi.org/10.1038/nn.3364
Pagán, C. N. (2022, November 28). Cognitive Behavioral Therapy (CBT) for Negative Thinking & Depression. Retrieved April 5, 2023, from https://www.webmd.com/depression/guide/cognitive-behavioral-therapy-for-depression
Nair, A., Rutledge, R. B., & Mason, L. (2020). Under the Hood: Using Computational Psychiatry to Make Psychological Therapies More Mechanism-Focused. Frontiers in Psychiatry, 11. https://www.frontiersin.org/articles/10.3389/fpsyt.2020.00140
Niv, Y., Hitchcock, P., Berwian, I. M., & Schoen, G. (2021). Toward Precision Cognitive Behavioral Therapy via Reinforcement Learning Theory (Ch. 12). In LM Williams and LM Hacks (Eds). Precision Psychiatry . American Psychiatric Association.
Montague, P. R., Dolan, R. J., Friston, K. J., & Dayan, P. (2012). Computational psychiatry. Trends in Cognitive Sciences, 16(1), 72–80. https://doi.org/10.1016/j.tics.2011.11.018
Comments